How to Create Error-Free Content With AI
Protect your brand—and your own butt—from the false facts and high-stakes hallucinations generated by AI.
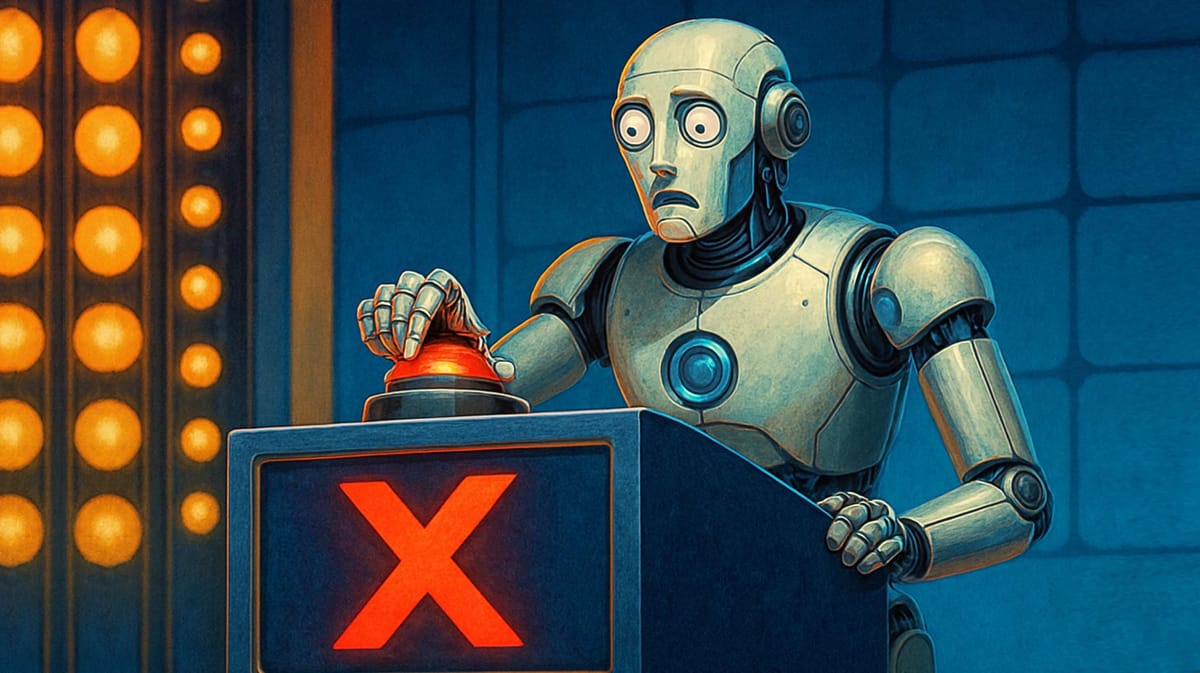
Be honest. How certain are you that your latest AI-assisted article, email, or ad campaign was 100% error-free? I'm not talking about typos. I'm talking about facts. Statistics. Quotes. Dates. Claims. AI is pumping out content at an astonishing pace, and much of it is riddled with inaccuracies. These falsehoods are creeping into blogs, news outlets, marketing campaigns, product manuals, and (not surprisingly) social media.
The risks are real. And waiting for AI to "get better" isn't an option. When Microsoft and Google suffer costly public gaffes because AI trips up, it can definitely happen to the rest of us. So, consider this a wakeup call. A primer on the state of AI errors. And how you can protect against them.
Oops.
Major brands have already learned the hard way. Microsoft laid off editorial staff after AI produced a travel article recommending an Ottawa food bank as a tourist attraction. Observers ridiculed the piece and questioned the lack of human oversight.
Meanwhile, Men’s Health magazine published an AI-generated piece that was supposedly fact checked, but experts discovered at least eighteen inaccuracies about low testosterone. The editors issued a public apology, but the damage to their credibility was done.
CNET quietly rolled out AI-written finance articles with factual errors in more than half of them, leading to major corrections and negative press.
A German publication printed an interview with legendary Formula One champion Michael Schumacher, who had been incapacitated since 2013 with a severe head injury. The interview was entirely fabricated by an AI, resulting in a major lawsuit.
And who can forget the embarrassing debut of Google's Bard chatbot, which produced a glaring error about the James Webb Space Telescope during a public demo, leading to a $100 billion drop in Alphabet’s market value.
These are not minor slip ups. They illustrate how one lapse in quality control can completely derail brand trust.
The Facts
The allure of AI generated marketing content is undeniable, but relying on these tools without rigorous oversight is playing with fire. Large Language Models (LLMs), the engines behind tools like ChatGPT, Claude, and Gemini, have a documented tendency to "hallucinate"—generating plausible-sounding but factually incorrect or entirely fabricated information.
This isn't an occasional glitch. Studies reveal significant error rates that vary wildly depending on the model and the specific task. For instance, one rigorous 2024 study examining the generation of academic references found GPT-4 hallucinated citations 28.6% of the time, while an earlier version of Google's Bard fabricated an alarming 91.4%.
Even newer models show issues, with a separate study finding Google's Gemini Advanced hallucinating financial citations at a rate of 76.7%. These figures underscore a critical reality: even the most advanced AI can confidently produce inaccuracies, posing a substantial risk to brand credibility when used unchecked for marketing content.
Furthermore, the idea of finding a single "best" or error-proof AI model is a misconception. Performance fluctuates significantly based on the task; a model excelling at creative writing might falter dramatically when asked for factual summaries or data points. While leading models like OpenAI's GPT series, Anthropic's Claude, Google's Gemini, and Meta's Llama continuously improve, none are immune to generating falsehoods, especially when dealing with complex, niche, or very recent information.
Perhaps most concerning of all, a 2025 McKinsey survey found only 27% of organizations using generative AI review all AI-generated content before external use. Yet research indicates LLMs struggle to reliably detect or correct their own errors. In other words, we know there are errors, but nobody's checking for them.
Truth or Consequences
One of the biggest risks, of course, is brand damage. Nobody wants to be a punching bag, but a single mistake can easily become fodder for social media, news outlets, and competitors. Then, there are the legal troubles. When misinformation leads to defamation lawsuits or false advertising claims, nobody cares if the AI wrote it. They only care who published it.
On top of that, once companies scramble to identify who is responsible, agencies, CMOs, and marketing teams can easily become scapegoats. Not exactly a recipe for healthy corporate culture. And, if this weren't enough, content deemed inaccurate or untrustworthy can swiftly lead to declines in domain authority and search ranking.
The big takeaway? Fallout from unintentional AI errors isn't limited to the 24-hour news cycle. The consequences can be widespread, long-lived, and hard to recover from.
Action Plan: How to Eliminate AI Errors
If you're starting to think an AI generated content catastrophe is inevitable ... you're not wrong. But only if you do nothing to prevent it. So, let's shift the focus to practical solutions you can implement right now. They build on each other and ensure that even if one method fails, another is there to safeguard against AI slip-ups.
Human Review is Non-Negotiable
A human editor or domain expert must always be involved. It should go without saying, but refusing to review AI output at all is simply inviting disaster. For starters, no machine can catch every nuance or subtle mistake.
Adversarial AI Fact Checks
When using an LLM to create content, ask it to reexamine its own output. Challenge it. Prompt it to verify any claims that lack sufficient proof. This self-interrogation can reveal where the AI is confused, winging it, or straight-up hallucinating.
Multi Model Consensus
Another approach is to cross-check content generated from one LLM with another. For example, a draft written with ChatGPT can be pasted into Perplexity with a prompt to look for suspicious stats, claims, and references. You'll be surprised how many things get flagged.
Retrieval Augmented Generation (RAG)
Instead of relying solely on an LLM's training data, RAG fetches information from specified, trusted sources, like an internal database or knowledgebase, to ensure more accurate and contextually relevant responses. Chatbots, content creation, and financial reporting are just a few prime use cases.
Citation and Plagiarism Tools
Copyscape, Scribbr, and similar platforms maintain extensive databases containing a wide range of text sources, including academic papers, books, websites, and other documents. They can detect blocks of borrowed text, sniff out bogus citations, and spot content that should be properly cited.
The Burden of Proof
In a future dominated by AI-driven content, credibility will be a precious commodity. The internet, already saturated with half-truths and dubious claims, faces an even steeper descent into mistrust with LLMs generating misinformation at scale. The technology’s productivity gains are undeniable, yet they magnify the risk of embedding falsehoods into public discourse. Marketers and brand leaders, in particular, bear the responsibility of ensuring their audiences are shielded from the pitfalls of unverifiable data. One fabricated quote, one inflated statistic, one inaccurate product detail can set off a chain reaction that tarnishes brand perception and widens the gap of distrust.
It's more than brand reputation at stake, though. As lazy, inaccurate, misleading content becomes more commonplace, the credibility of entire platforms and channels could be called into question. Audiences have grown savvy, skeptical, and increasingly intolerant of anything that reeks of carelessness. And that isn't going away anytime soon. If we're not careful, the old saying "Don't believe everything you read on the internet" will turn into "Don't believe anything you read on the internet." Let's make sure that doesn't happen.