The Biggest Obstacle to AI Adoption? Marketers.
Let's stop talking about what AI can do—and start focusing on how to do it.
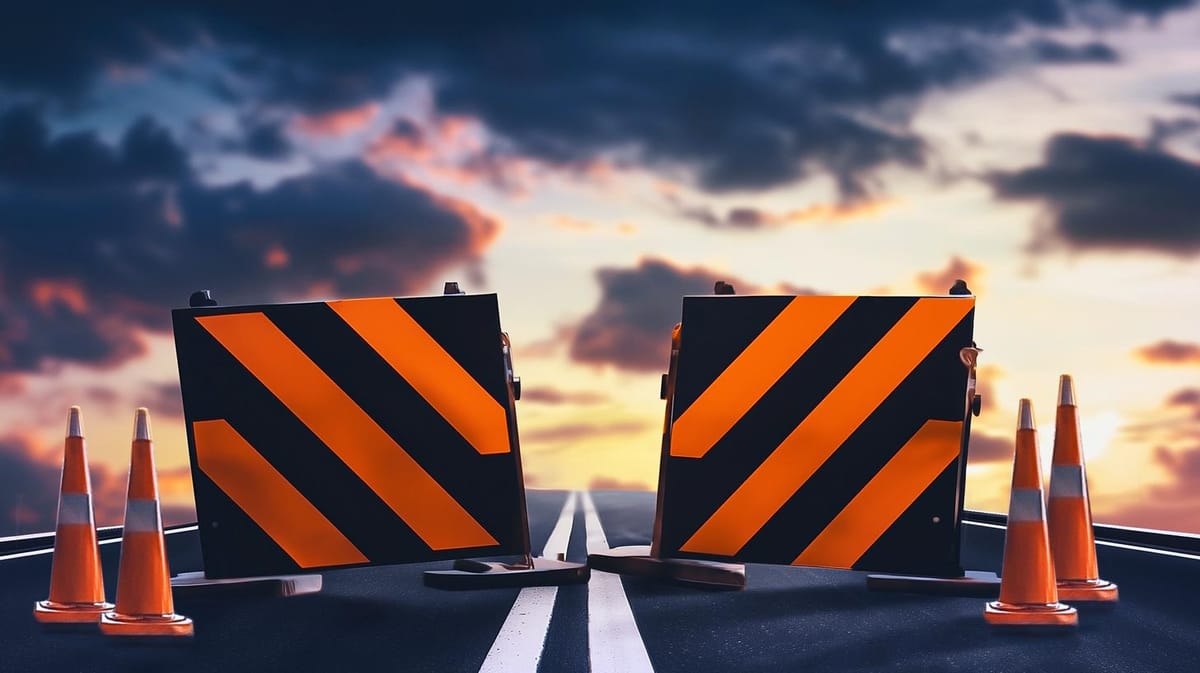
There is a moment I keep returning to in my mind. It happened during a recent “AI for Marketers” course I enrolled in—one of those free online sessions where you expect just enough depth to make it worth your while, or at least worth your email address. Midway through, I realized something strange. The course had shockingly little educational value. It was more like an ad for artificial intelligence. "With AI, you can predict churn. You can deliver personalized experiences at scale. You can automate everything. It slices, it dices..."
I think I speak for all the attendees when I say, "Great! Now what?" The benefits, use cases, and adopt-or-get-left-behind urgency were all there. But not a single word addressed even the first step.
That moment didn't let go of me. It felt like a perfect microcosm of what we, as marketers, have done to ourselves. We are professional hype creators, finely tuned to stoke desire around shiny new things. But in our rush to tout the wonders of AI, we’ve managed to omit the instruction manual. In other words, we’ve become our own obstacle to adoption. We’ve seduced our industry with the shimmering promise of “what” AI can do ... while blatantly ignoring the all-important “how” to do it.
I want to talk about that “how” because, deep down, it’s the piece everyone needs. In practice, marketing teams are asking the same questions with every AI use case:
Is there an app for that?
Do I need to hire a developer?
Can I do it on my own with ChatGPT?
These are the questions marketers (and AI courses) routinely fail to answer. So, I'm doing it here. There are three ways to adopt AI in marketing, each corresponding to the questions above. Each distinctly suited to different ambitions, budgets, and constraints. Consider this a framework for evaluating an AI use case and choosing a path for implementation. Consider this the "how."
1. The Self-Service Path
If you're one of the nine zillion people who've experimented with ChatGPT, you're already familiar with this path. You prompt a chatbot to generate a quick draft of a sales email, a blog article, or maybe a product description, and marvel at how coherent it sounds on the first try. This is the entry-level magic of large language models: they can pull from vast amounts of online text to produce a surprisingly polished result. Marketers often feel they’ve stumbled onto a secret weapon, discovering that routine tasks such as brainstorming article topics or clustering user feedback can be accomplished in seconds. The sense of speed is intoxicating, and it’s often enough to get even old-school marketers over that initial AI hurdle—no more dreaded blank-page syndrome.
The Caveat
Large language models like ChatGPT, Claude, and Gemini are prone to inventing facts, fabricating references, or exhibiting biases that might clash with your brand's values. With the self-service route, you trade off scale and advanced capabilities for immediacy and low cost. A marketer can do a lot on their own with a free or low-cost LLM, but the tool’s potential is only as good as the data and prompts fed to it. Use it to ideate subject lines or short-form copy, and you might get gold. Lean on it for flawless research or data analysis, and you might get nonsense. These shortcomings are why many marketers gravitate toward what I call the “off-the-shelf path.”
2. The Off-the-Shelf Path
If the self-service path is the shallow end, off-the-shelf AI represents a broader pool where most marketing teams will end up swimming. This approach involves using an existing application—often a SaaS product—that embeds AI under the hood. Think specialized “wrapper” solutions built on top of GPT-4 (or another engine) but laser-focused on particular tasks, like SEO or text-to-video generation. Want an automated note taker for meetings? Try Fireflies.ai. Need an all-purpose content marketing assistant? Jasper can help. No prompt engineering required, you just let the application work its magic every time.
The “Wrapper” Revolution
Silicon Valley investors are pouring billions into AI startups because they see a future where non-technical marketers can do extraordinary tasks without hiring a data scientist. These wrapper solutions provide intuitive solutions to very specific problems—say, advanced audience segmentation—without requiring you to know anything about model architecture or API calls. For many, it’s a sweet spot: you get a quick and tangible way to harness the power of AI without the overhead of building it all yourself. The trade-off, of course, is that you’re at the mercy of the vendor’s roadmap and pricing, and your data might live on a platform you can’t fully control. Still, for standard marketing challenges—campaign optimization, competitive intelligence, content automation—this approach can deliver tangible value.
Off-the-shelf tools are arguably where AI will flourish in marketing over the next few years. Many liken it to the “app store” phenomenon, predicting that we'll tire of experimenting with ChatGPT for every need and choose to rely on a stack of low-cost, turnkey AI tools instead.
3. The Developer Path
Some organizations, however, have ambitions or complexities that go beyond anything an off-the-shelf solution can handle. Maybe you collect highly specific user data that calls for a bespoke predictive model. Maybe you want AI to interface with your internal knowledge base, your CRM, and your custom product usage logs. Or perhaps you see a chance for a powerful competitive edge—one that can only emerge from a model tailored exactly to your needs. In these scenarios, you move toward the fully custom approach, which involves building your own AI system from scratch.
Understanding the AI Developer Landscape
Many people assume “AI developer” is a singular role, but in practice, several specialized skills come into play. Data scientists wrangle data, define the model architecture, and figure out how to train it. ML engineers handle model development, training, and deployment. MLOps specialists oversee the maintenance of these models, because like any system, models degrade if not regularly updated with fresh data. And you may still need a separate integration developer or team to stitch your new AI capabilities into the rest of your marketing stack. That’s a lot of talent—and it comes at a cost.
For large brands or those with very particular needs, the reward can be huge. A custom AI engine that truly understands your user base, your product quirks, and your business rules might be a game changer. But it also means you’re committing to a long-term relationship with data science, from the early build to the ongoing upkeep. Some projects take six months to a year just to reach a functioning MVP. Others end up in “proof of concept” purgatory, never fully launched because of shifting business priorities.
That Wasn't So Hard, Was It?
The irony, as I see it, is that we marketers managed to hype AI so effectively we forgot to provide a proper roadmap—even for ourselves. Once you recognize that gap, though, something important shifts. You begin to understand the difference between flashy promises—“AI can predict churn”—and the practical realities of implementation.
You ask whether your team is better off tackling a use case with ChatGPT or investing in an app that can accelerate time to value. You wonder if your data is so unique that you need in-house developers to engineer a solution.
None of these questions are trivial. But if we want to truly adopt AI and not just admire it, we have to answer them. We have to stand at the fork in the road—DIY, off-the-shelf, or custom—and commit to one. My hope is that, by acknowledging the "how," you’ll find your path with less second-guessing and fewer false starts. Because there’s no shortage of ways AI can dazzle us all. It’s the implementation counts.